AI in Marketing: Hype vs. Reality for CMOs
Discover the real impact of AI in marketing with this in-depth guide for CMOs. Learn what’s hype, what works, and how to drive ROI with proven AI strategies, use cases, and case studies.
Marketing
7
min read
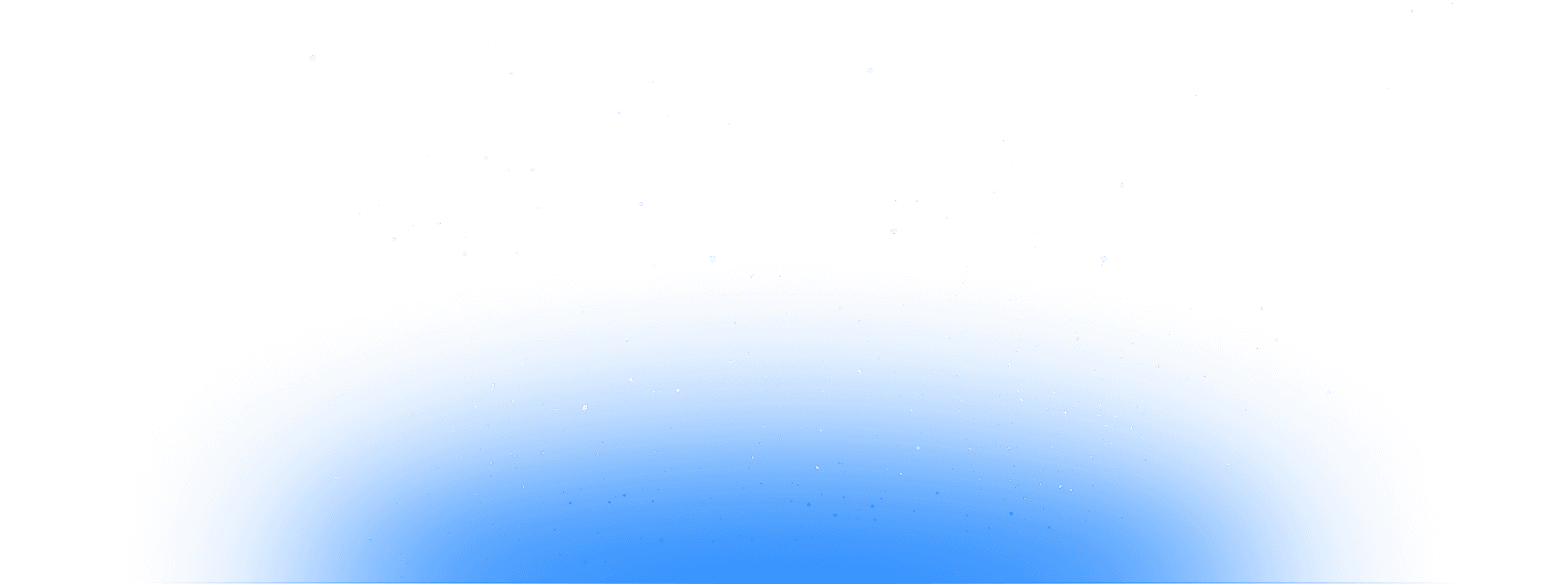

Artificial intelligence (AI) in digital marketing is surrounded by sky-high expectations – every headline and vendor pitch seems to promise a marketing revolution. The global AI market is valued at over $196 billion and is projected to grow 13-fold in the next seven years. Unsurprisingly, CEOs and boards are pressuring marketing leaders to “get on the AI bandwagon” to stay ahead of the curve. But many companies are rushing to adopt AI, even “AI-washing” their products with inflated claims, while marketers quietly worry about what is real versus what is just hype. Several years into the hype wave, many CMOs feel that their AI investments have yet to yield tangible performance gains.
This article will explore why AI has been touted as a game-changer (and what’s driving that hype), do a reality check on where AI genuinely excels (and where it struggles), highlight key AI marketing use cases with proven ROI metrics, and share real marketing AI case studies. Finally, we will explain Agility’s own pragmatic approach, using machine learning to connect precision brand advertising with business outcomes.
The goal is to give enterprise CMOs and marketing VPs a clear, insight-driven perspective on AI marketing strategy: what works today, what’s overblown, and how to strategically leverage AI for marketing ROI with real results, not just promises.
Drivers of the AI Marketing Hype
What’s feeding the frenzy around AI in marketing? Several forces are at play:
Media Buzz and Big Promises
Breakthroughs like generative AI (e.g., ChatGPT, Jasper, Claude, Gemini, or Grok) have dominated headlines, fueling the narrative that AI will transform marketing overnight. Tech prognosticators tout bold claims like “hyper-personalization of every customer interaction” and “predicting consumer behavior with pinpoint accuracy.” This constant drumbeat builds C-suite FOMO (fear of missing out) on the “next big thing.” Reports show that investors also reward the hype—startups merely mentioning “AI” attract 15–50% more funding than others. The result is enormous pressure on marketing leaders not to be left behind in what feels like a gold rush.
Vendor Narratives and AI-Washing
Marketing technology vendors have seized on the AI trend, branding a wide range of software as “AI-powered.” In reality, many of these tools still rely on basic algorithms or rule-based automation. This AI-washing, or making exaggerated or false claims about AI capabilities, is rampant. For example, traditional analytics or automation products are often relabeled as “AI-driven” to capitalize on the hype. Such vendor marketing creates inflated expectations among buyers. CMOs are bombarded with pitches that promise AI magic (with minimal proof), making it hard to separate true innovation from buzzwords.
C‑Suite and Boardroom Pressure
With AI in the media spotlight, CEOs and boards ask CMOs, “What’s our AI strategy?” The C-suite often views AI as a strategic priority for achieving competitive advantage, and many CEOs are pushing their organizations to adopt AI faster than teams may be comfortable or capable. This top-down pressure means CMOs feel compelled to demonstrate progress on AI adoption, sometimes before the technology or data foundation is fully in place. In Gartner’s CMO survey, 73% of high-performing marketing organizations are already using generative AI in some form, whereas 27% of CMOs admit their teams have only limited or no AI deployment so far. In other words, the leaders are leaning in, and those who haven’t started worry about being at risk of falling behind. This dynamic can prompt marketing teams to experiment with AI, even if the practical ROI has not yet been proven.
Savvy CMOs know that beyond the flashy demos and bold promises, the real question is performance. What tangible outcomes can AI deliver? To answer that, we need a reality check on AI’s true capabilities in marketing today.
Where AI Excels vs. Where It Falls Short
AI is undeniably making inroads in marketing, but it’s not a magic wand. The reality is that AI offers powerful tools in certain areas, while still struggling or requiring heavy lifting in others. Here’s the balanced view:
Where AI Is Delivering Value
AI truly excels at data-crunching and pattern recognition at scale, which lends itself to several marketing applications that drive results:
Personalization and Targeting
Machine learning algorithms can analyze vast datasets, including transactions, browsing behavior, demographics, and more, to segment audiences and personalize content far more effectively than manual methods. This has led to more relevant, tailored campaigns. Is it perfect hyper-personalization? Not always, but AI-driven targeting is demonstrably more precise than one-size-fits-all marketing. For example, the data shows that AI-based targeting can significantly boost conversion rates. One study found that companies using AI for marketing saw a 25% increase in conversion rates compared to traditional methods. AI is particularly adept at optimizing who receives which offer, when, and on which channel, in ways that humans would struggle to compute.
Predictive Analytics and Decision Support
AI’s number-crunching power also shines in forecasting and analysis. Predictive analytics models can sift through historical data to predict outcomes like churn risk, lifetime value, or purchase propensity. This isn’t a crystal ball, as marketing will always have its share of unpredictability, but it’s a better forecast for the marketing landscape. By spotting patterns (e.g., which behaviors signal a customer is about to drop off), AI helps marketers make more informed decisions. It excels at answering questions like “Who is likely to convert?”, “What products will this segment be interested in next?” Armed with those insights, marketers can proactively tailor strategies (for instance, targeting high-risk customers with retention offers before they churn). The bottom line is better anticipation of customer needs and market shifts, resulting in a more informed strategy.
Media Optimization and Efficiency
Another area of real impact is using AI to optimize media spend and channel mix. AI can ingest campaign data across digital channels and automatically adjust bids, budgets, and placements in real time for maximum performance. AI drives efficiency by iteratively testing and learning the best allocation of dollars, which used to require weeks of manual analysis. Marketers using AI-powered ad platforms have seen tangible benefits, such as 30% lower customer acquisition costs (CAC) through automated bidding. AI can also identify wasteful spending and eliminate it quickly. The result is leaner, more precise media spending. Deloitte found that companies utilizing AI for ad optimization achieved an average 22% improvement in marketing return on investment (ROI) across campaigns.
Where AI Struggles (for Now)
Despite those strengths, AI has its limitations and implementation challenges in marketing:
Data Quality and Integration
The mantra “garbage in, garbage out” applies strongly to AI. AI models won't perform well if your customer data is siloed, riddled with duplicates, or even just scarce. Many marketing organizations grapple with fragmented data across their CRM, web analytics, social media, and sales systems. Cleaning and unifying this data is tedious but essential work that must be done before AI can add value. AI also needs sufficient training data – for a niche brand with limited historical campaigns, fancy algorithms may not have enough examples to learn from. In reality, a significant amount of time spent on AI projects is dedicated to data preparation and integration. CMOs must ensure their data foundation is solid since even the best AI tool will underwhelm otherwise. Notably, a recent Gartner finding revealed that over a quarter of marketing leaders saw little to no benefit from generative AI in areas such as cost reduction or customer service, partly because messy data and processes hinder AI's ability to deliver tangible savings. Robust data hygiene and infrastructure remain essential prerequisites for achieving AI success.
Creative Strategy and Content Nuance
AI can automate and augment, but it struggles with creativity and brand nuance. Today’s AI, even creative generative models, can churn out basic copywriting, product descriptions, or variations of ads, but it lacks the true creative spark and contextual understanding that human marketers provide. AI-generated content tends to be derivative since it’s based on patterns in past data, rather than original insights. It can also miss subtle brand voice elements or cultural sensitivities. As one analyst quipped, AI content is more of a “digital court jester” than a “content king,” improving, but lacking the human touch. For strategic campaign concepts, storytelling, and big creative ideas, human marketers remain irreplaceable. Successful teams pair AI with human insight, for instance, using AI to draft a report or test lots of ad variants, but having marketers curate and refine the final creative. Relying on AI alone for content marketing strategy or creative direction is a recipe for generic output that may miss the mark with audiences.
Integration and Skill Gaps
Implementing AI in the marketing stack isn’t a plug-and-play process. Often, AI capabilities need to be integrated into existing marketing platforms or workflows, which can be a technically complex task. There may be a need for new tools, APIs, or cloud services, and for ensuring different systems (ad platforms, CRM, analytics) feed data into the AI engine. Many organizations also face a talent gap, requiring advanced analytics and data science skills to tune AI models or even to interpret their outputs. Without the right people and process changes, AI initiatives can stall. There’s also change management: your team must trust and adopt the AI’s recommendations. If the organization isn’t culturally ready to act on machine insights, then even the best AI solution won’t get utilized. It’s telling that only about 15% of CMOs in one survey reported “broad use” of AI across their marketing team, with most still in pilot phases, figuring out how to fit AI into the puzzle. AI technology may be advanced, but organizational readiness often lags.
The bottom line: AI is not a magic button that instantly transforms marketing, but it is a potent accelerator when applied to the right problems under the right conditions. The reality is that AI can deliver exceptional personalization, predictive insights, and efficiency gains, as proven in numerous use cases. Still, it requires good data, a clear strategy, and human oversight to reach its potential. Next, let’s explore some of the high-impact use cases and the metrics that demonstrate AI’s tangible impact on marketing ROI, efficiency, and customer experience.
Key AI Marketing Use Cases and Metrics for ROI, Efficiency, and CX
To cut through abstract promises, it helps to look at concrete use cases of AI in marketing and how we can measure success. Below are some of the most effective AI marketing applications today, along with key metrics and outcomes that matter to CMOs:
Personalization and Customer Experience Improvements
One of the top priorities for AI marketing strategies is delivering personalized customer experiences at scale. Machine learning models can analyze customer data to tailor product recommendations, messages, and offers to each individual's specific needs. In practice, AI-driven personalization has shown impressive results. Adidas, for example, used AI to personalize digital ads and saw a 30% increase in conversion rates across campaigns. Similarly, Starbucks’ AI-powered recommendation engine, which analyzes over 400,000 variations of offers, achieved a 3× increase in customer response rates by serving more relevant and timely offers. These metrics (conversion lift and response rate) translate directly to higher sales and a better user experience, as customers feel the brand understands their needs. When measuring ROI with AI personalization, CMOs should track improvements in conversion and retention specifically attributable to those AI-driven interactions, as well as changes in customer lifetime value resulting from more engaging, personalized marketing.
Predictive Analytics & Customer Insights
AI’s predictive capabilities help marketers move from reactive to proactive. Use cases include lead scoring (predicting which prospects are most likely to convert), churn prediction (identifying customers at risk of leaving), and demand forecasting (anticipating product demand or campaign performance). Success metrics: Uplift in retention or acquisition due to acting on predictions, value of prevented churn, and accuracy of forecasts. For instance, Netflix’s recommendation algorithm is credited with saving an estimated $1 billion annually by reducing subscriber churn through better content suggestions. While not a traditional ad campaign, it showcases AI’s impact on customer retention (CX) and revenue. Marketers leveraging predictive models might similarly measure how much an AI-driven churn model improves retention rates or how lead scoring models increase conversion of top-tier leads. The key is to compare outcomes before and after AI insights are applied. If your predictive analytics program can identify high-value customer segments that respond 20% more to a tailored offer, that percentage lift and the revenue it brings become the ROI metrics for the AI effort. Many organizations are achieving double-digit improvements in campaign performance by acting on AI-driven insights. For example, one McKinsey study noted that average conversion rates improved by approximately 25% when AI was applied to marketing and sales decisions. Those are the kinds of numbers that make a bottom-line difference.
Media Spend Optimization & Marketing ROI
AI is highly effective at optimizing media buying and channel allocation – essentially, making each marketing dollar work more efficiently. Use cases include programmatic ad bidding using AI (like Google’s Smart Bidding), budget allocation across channels, and even marketing mix modeling with machine learning. Success metrics: Return on ad spend (ROAS), cost per acquisition (CPA), and overall marketing ROI. Google reports that advertisers using its AI-driven bidding see an average 30% reduction in CPA (cost per acquisition) compared to manual bidding, meaning a significantly lower price to acquire a customer. As mentioned earlier, Deloitte found a 22% average increase in marketing ROI when companies applied AI to optimize their advertising investments. These improvements stem from AI’s ability to reallocate spend to the best-performing audiences and channels continuously, and to pause or adjust underperforming tactics in real-time. For CMOs, the key metrics to watch are marketing ROI with AI versus without. If your traditional campaign had a 3:1 ROAS and after implementing AI-driven optimization, it’s 4:1, the delta represents AI’s contribution. Tracking year-over-year efficiency gains, such as a decrease in cost per lead and cost per sale, is another way to quantify impact. The evidence so far shows AI can substantially increase marketing efficiency and effectiveness, directly improving the ROI of marketing campaigns.
Marketing Automation and Real-Time Adaptation
AI also powers automation use cases that improve operational efficiency and agility. This ranges from automated content generation (e.g. drafting product descriptions or basic emails) to chatbots handling customer inquiries, and real-time analytics that adjust campaigns on the fly. A great example is real-time campaign optimization, with brands using AI-based analytics able to adapt to trends 5× faster on average than those using traditional manual analytics. A faster reaction means capitalizing on opportunities (or resolving issues) in hours instead of days, which can be the difference between riding a meme wave or missing it. Starbucks’ real-time offer personalization is a case in point–the system adjusts in the moment and drives significantly higher engagement than static campaigns. On the automation front, AI chatbots and recommendation engines enhance the customer support experience by providing instant responses or suggestions, measured by customer satisfaction and reduced response times. Internally, automating repetitive tasks (such as reporting, data analysis, and content drafts) frees up staff hours. A key metric might be the monthly analyst hours saved, which can be redeployed to higher-value work. While harder to quantify, one can also measure content output; for example, some marketing teams have used generative AI to produce 2x more content pieces in a given time, helping meet growing content demand (notably, marketing content needs rose 1.5× in 2023, and AI is seen as a solution to fill that gap efficiently). Ultimately, the value of AI-driven automation shows up in both cost efficiency and the ability to deliver a better, more responsive customer experience.
Each of these use cases ties AI activity to metrics CMOs care about like conversions, retention, ROI, speed, and customer satisfaction. By defining clear KPIs at the start (e.g., “increase email open rate by X% with AI-driven send-time optimization” or “reduce churn by Y% with predictive modeling”), marketing leaders can objectively evaluate AI’s impact.
Agility’s Approach: Using Machine Learning to Connect Media Precision with Business Outcomes
Amid the hype, Agility has taken a deliberate, outcomes-focused approach to applying machine learning in marketing. We recognize that AI is not a magical, all-in-one marketing solution, but rather a tool to enhance decision-making and drive performance when applied to the right problems. Agility’s philosophy is to use machine learning as a means to an end: optimizing media precision to achieve tangible business outcomes, such as revenue, ROI, and growth, rather than as a shiny object. In practice, this means we don’t position Agility as a full-blown “AI platform” that replaces marketers. Instead, we embed powerful ML algorithms and AI-based processes under the hood of our marketing analytics and execution workflows to make them more innovative and precise.
How Agility uses AI and ML:
Enhanced Targeting and Segmentation
We utilize AI to help identify high-value persona segments and determine the optimal campaign targeting criteria. This ensures media spend is directed with pinpoint precision, focusing on the right customers on the right channels. Our clients benefit from more efficient campaigns that target those most likely to convert, thereby incrementally improving key metrics such as conversion rate and cost per acquisition.
Optimizing Channel Mix and Budget Allocation
A core strength of Agility’s approach is using data to connect each marketing touchpoint to business outcomes, such as sales or leads, and then optimizing the media mix accordingly. Our machine learning models perform multi-channel attribution, determining the contribution of each channel and campaign to a conversion. With this attribution data, we can then automatically reallocate budgets to the best-performing channels or tactics. For example, suppose the ML attribution analysis indicates that paid social is yielding a higher ROI than expected, while a display campaign underperforms. In that case, the system can suggest reallocating spend to paid social to maximize returns. By continuously crunching the numbers, the AI ensures media precision – every dollar is placed where it has the greatest impact on outcomes. This approach directly drives marketing ROI with AI, as evidenced by industry benchmarks, including a 22% boost in ROI from AI-based media optimization. Agility’s clients see this in practice through improved return on ad spend and lower waste in their budgets.
Iterative Creative Production
Agility’s in-house precision creative team helps our clients save time and money by offering rapid iterative creative production and testing an extensive library of creative variants to identify the right messaging and creative for each persona audience. AI helps to speed up the production of so many ad variants while providing valuable insights for future iterations. Clients using Agility’s precision creative services have seen performance improvements of up to 73% compared to their own assets.
In summary, Agility’s approach involves augmenting marketing teams with machine learning to enhance precision and performance. We focus on proven applications – targeting, channel optimization, and attribution – that directly influence marketing ROI and efficiency. We continually loop back to results: our machine learning recommendations are validated by actual lift in key metrics (conversion, ROI, etc.), not just model scores. By taking this focused, outcome-oriented path, Agility helps brands cut through the AI hype and achieve the tangible benefits of AI in their digital marketing efforts. Our clients gain the advantages of AI-powered tools like smarter insights, more effective spend allocation, and faster optimizations without sacrificing the strategic human element. The outcome is marketing programs that are both data-driven and agile, connecting every media dollar to business growth in a clear, demonstrable way.
Conclusion
The rise of AI in marketing is both exciting and daunting for today’s CMOs. On the one hand, the hype promises a future of autonomous campaigns and science fiction-like personalization. On the other hand, the reality today is that AI is a powerful enabler in specific areas, delivering significant improvements in performance and efficiency; however, it requires a strategic approach, high-quality data, and human oversight to truly yield its benefits. Enterprise marketing leaders should approach AI with a balanced mindset: be optimistic about the proven use cases and outcomes, but stay grounded by setting realistic goals and demanding evidence of ROI. The examples we’ve discussed, from Adidas’s conversion boost to Starbucks’s engagement win, demonstrate that AI is already driving marketing ROI when applied smartly. At the same time, the pitfalls (from data issues to overhyped tools) remind us that AI is a means to an end, not a magic bullet.
For CMOs, cutting through the noise means asking the right questions: Will this AI initiative improve a key metric that matters to our business? How will we measure success? Do we have the data and team in place to support it? By focusing on the strategic use of AI in the service of precision and better outcomes, you can turn the hype into real value. Agility’s mission is to partner with brands on exactly that journey.
Ultimately, AI in marketing should not be adopted simply because “everyone’s doing it,” but rather because it can drive better business results. The technology is a powerful tool in the CMO toolkit, and those who wield it wisely will indeed gain an edge in marketing ROI, customer experience, and growth.
FAQs
What are the most effective AI use cases in marketing today?
Several AI applications have proven especially effective for marketers. Personalization is a top use case, using AI to tailor content or product recommendations to individuals has yielded significant conversion gains (for example, Adidas saw a 30% increase in conversions from AI-driven ad personalization). Predictive analytics is another approach, where AI models predict customer behavior (such as churn or next-best product) so marketers can act proactively. This has improved retention and customer lifetime value. For instance, Netflix’s AI recommendations have reduced churn, saving an estimated $1 billion, illustrating the impact of predictive personalization. Media and ad optimization is a third high-impact area – AI can automatically adjust bids and budgets to maximize performance, often lowering customer acquisition costs and boosting ROI. Companies that utilize AI for ad spend optimization achieved a 22% higher marketing ROI in one study. Other effective use cases include AI-driven segmentation (identifying high-potential customer segments), chatbots for customer service (enhancing response times and freeing human representatives for more complex issues), and marketing automation (automating email campaigns, basic content creation, and other marketing tasks to increase efficiency). The common thread is that these use cases either increase revenue (through better targeting and personalization) or decrease costs (through automation and optimization) – or both – making them valuable for marketing ROI.
How can CMOs measure ROI from AI-powered marketing tools?
Measuring ROI for AI in marketing should be approached like any other marketing investment – by tying the tool’s performance to specific, measurable metrics and tangible business outcomes. First, define the specific KPI the AI tool is expected to influence (e.g., conversion rate, click-through rate, cost per acquisition, retention rate, etc.). Then set a baseline using historical data or control groups. For example, if you implement an AI personalization engine on your website, compare the conversion rate or average order value for the target audience that sees AI-personalized content with that of a control group that sees standard content. Any improvement in those metrics can be attributed to the AI, provided all other factors remain constant. Financially, calculate the additional revenue generated or costs saved as a result of that lift. Suppose AI-driven targeting improves conversion rates from 2% to 2.5% – that 0.5% increase (which is a 25% relative lift) can be translated into extra revenue per campaign. Similarly, if an AI bidding tool lowers your cost per acquisition from $50 to $35 (a 30% reduction), you can calculate how many dollars are saved for every customer acquired. CMOs should also account for the investment cost in the AI tool and any implementation services – the net ROI will be (financial gain from AI – cost of AI) / cost of AI * 100%. It is essential to measure over a meaningful period and with a sufficient sample size to account for variability. In practice, many CMOs conduct pilot tests: they introduce the AI in one region or channel, keep another as a control, and measure the incremental lift. Metrics such as ROAS (Return on Ad Spend), incremental sales, customer lifetime value uplift, or time saved (which can be converted to cost savings) are all ways to quantify the ROI. Regular reporting and dashboards that attribute outcomes to the AI tool help maintain clarity and transparency. Ultimately, an AI initiative should demonstrate a positive return on investment (ROI) by either boosting revenue, reducing costs, or improving efficiency sufficiently to outweigh its expenses. If it doesn’t, then it may be a sign to recalibrate or try a different application.
How does Agility use AI to improve marketing outcomes?
Agility utilizes machine learning in a highly focused manner to deliver improved marketing outcomes for our clients. We embed machine learning modules into key parts of the marketing process. One major area is media optimization: Agility’s platform uses ML algorithms to analyze campaign performance data and automatically adjust the channel mix and budget allocations for maximum performance. It identifies which channels, ads, and audience segments are yielding the best outcomes (e.g., conversions or revenue). It reallocates spend toward what works and away from what doesn’t, in near real-time. This boosts efficiency, yielding more results for the same spend. We also use AI for persona targeting development, processing large datasets (including customer demographics, behaviors, and context) to identify high-potential audiences and personalize messages to them. This improves response and engagement metrics by ensuring the right people get the right content. Finally, Agility uses AI to increase the speed of creative production in an effort to test into certainty. Importantly, all of Agility’s AI use is geared towards augmenting the marketing team, providing data-driven recommendations and automated optimizations, while leaving final strategic decisions with the marketers. The result is that Agility’s clients achieve better marketing ROI, lower acquisition costs, and improved growth metrics, powered by AI insights behind the scenes. In short, we use AI as the intelligent engine that makes marketing campaigns more precise and accountable to business results, allowing CMOs to make informed decisions that improve performance.
Share in...